Business Brief
Future-Proofing Evidence for Better Patient OutcomesExecutive Summary
In the medical device and pharmaceutical industries, data harmonization has been applied in adverse event tracking from post-market data, complaint analysis, and clinical study data. However, these harmonization efforts are often siloed within functional areas, with each categorizing the data according to their own methodology. To combat these issues of hidden, scattered, or siloed data, organizations are increasingly creating data harmonization governance committees to implement a common data structure across the product lifecycle, including for systematic literature reviews and RWE. This allows data from all sources to be easily combined into a comprehensive analysis package that can be understood and consistently interpreted across the organization, which in turn allows the right data to be accessible to the right decision-makers at the right time.
The result is that critical decisions can be made quickly, decreasing cost and enabling better resource allocation. By contrast, lack of data harmonization means increased potential for regulatory rejection, with its related costs to both the company and the patient community waiting on the product.
Download the PDF of this Brief
Introduction
The concept of data harmonization in healthcare is not new. In 2016, the FAIR (Findability, Accessibility, Interoperability, and Reusability) Guiding Principles for scientific data management were established across academia, industry, funding agencies, and publishers in order to improve the infrastructure supporting the reuse of scholarly data. The goals of the guidelines were to support clinical and scientific data reuse and to enhance the ability of machines and artificial intelligence to find and synthesize data. The FAIR principles form the basis for data harmonization, namely, that information can be found easily by virtue of the standardization of metadata, that the data is accessible to multiple functions or groups, that all functions understand the standardization language and its knowledge representation, and that the data is reusable throughout the organization. These principles were applied to several publicly available data sources, including the Harvard Dataverse, in projects that immediately demonstrated the value of having data that was accessible to and shareable by the research community.1
The FAIR principles are easily transferable to organizations that need seamless data exchange across the product lifecycle and between functional areas.
Pharmaceutical and MedTech organizations, in particular, benefit from data harmonization, including in their systematic literature reviews, both during product development and in the submission and review process. Systematic literature reviews can contain a tremendous amount of critical data, especially on well-established products.
Searches can contain hundreds of relevant papers and tens of thousands of patients between pivotal, supporting, and contextual literature. RWE databases or platforms that curate RWE, such as Omnihealth Group, contain millions of data points that increasingly need to be integrated into safety and performance outcomes. Regulatory agencies want to see that product data is organized and accessible and that it includes data from systematic literature reviews and RWE on the product under evaluation or on like products. Harmonized data is easier to integrate and aggregate, which is essential for creating comprehensive and standardized evidence repositories. In turn, this integration allows for the combination of data from multiple studies, enhancing the breadth and depth of the curated evidence and ensuring that submissions to the food and drug administration (FDA), European medicines agency (EMA), and other regulators are complete, consistent, and successful in meeting all necessary requirements, thus reducing delays and rejections.
Compliance with global data standards thus facilitates faster and smoother market access and approval in different regions. Evidently, the ultimate objective of data harmonization in healthcare is to enhance patient outcomes, both by facilitating more informed medical decisions and by driving medical research and innovation.
The improved accuracy of data, along with the ability to analyze all the consolidated data, has the potential to minimize the risk of adverse events and enhance patient safety. Predictive analytics can also detect trends in a data package that may not have been noticed if the data was siloed. Finally, by unifying and harmonizing the ontology and metadata, harmonization future-proofs the data, making it ready for advanced analytic and AI applications.2,3
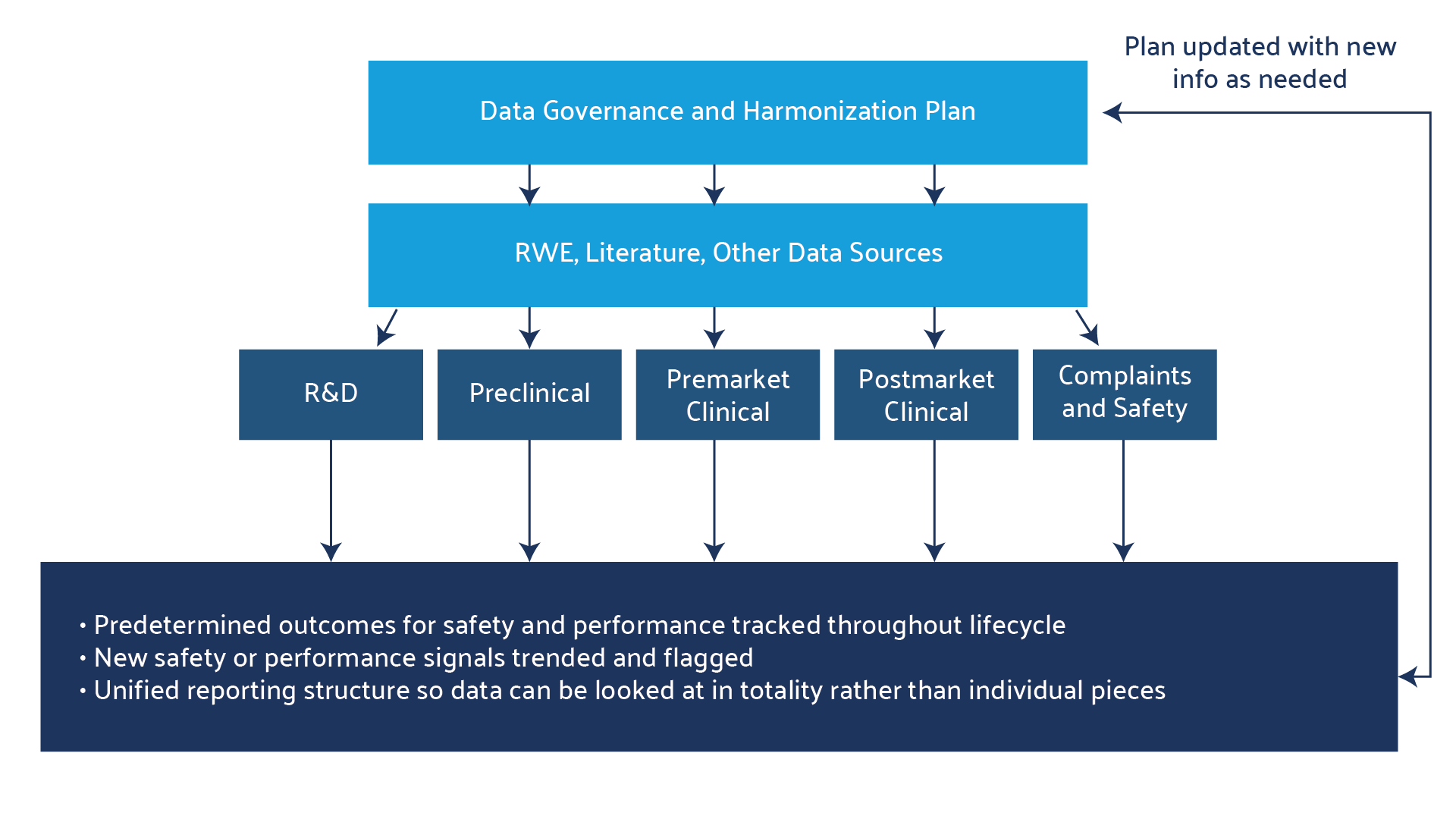
Data Harmonization Governance — the Essential Foundation
A data harmonization governance committee or working group is a critical cross-functional team that sets the stage for a successful harmonization process. The creation of such a team is the foundational step toward establishing the policies, roles, standards, and ontology for organizing data.
Implementing a data harmonization project requires a structured approach to managing and ensuring the quality, availability, integrity, and security of data within a healthcare organization.3
Implementing a Data Governance Framework
Data harmonization is not simply the compiling of data in a single database or drive. At the outset, the groundwork needs to be laid as to what the goals and endpoints of the project are, and all stakeholders in the plan should be engaged to ensure buy-in.4 This is why establishing a governance committee is essential. This committee should work through all the steps below before executing on any harmonization plan.4
- Define goals: Clearly define the goals and objectives of your data governance initiative, such as improved data quality, compliance, and operational efficiency.
- Form a cross-functional governance team: Establish a governance team that spans functional silos, comprising representatives from IT, digital transformation, clinical/medical affairs, regulatory, legal, and other relevant departments. This team will provide diverse perspectives and ensure alignment with organizational goals.
- Set governance policies, roles, and responsibilities: Develop and document data governance policies that outline the rules, guidelines, and procedures for managing data across its lifecycle. Policies should cover data access, usage, quality standards, security measures, and compliance requirements. Roles may include data stewards, who are responsible for data management and quality, and data owners, who are responsible for the raw data and assets.
- Create a comprehensive inventory of data assets: Conduct a thorough inventory of all data assets within the organization. These assets include complaints, post-market surveillance, clinical study data, and systematic literature review analysis. Identify all the data sources, formats, and locations along with the stakeholders involved in managing each dataset.
- Classify and categorize data: Classify data based on sensitivity, regulatory requirements, and business value. This classification helps prioritize data governance efforts and determine access and protection measures. Data can then categorized through a uniform industry ontology or common clinical elements so that they can be universally analyzed and understood and to enable data organization and easy searchability. At this point, plans for both retrospective and proactive harmonization should be made as well.
- Develop data integrity standards: Define data integrity standards and metrics to measure data accuracy, completeness, consistency, and timeliness.
Establish processes for data validation, cleansing, and the maintenance of data hygiene to ensure consistently high-quality data. - Implement data security measures: Implement robust data security measures to protect data from unauthorized access, breaches, and cyber threats. This includes encryption, access control, authentication mechanisms, and regular security audits.
- Ensure regulatory compliance: Ensure compliance with relevant regulations, for example, general data protection regulation (GDPR), health insurance portability and accountability act (HIPAA), and internal policies through regular audits, documentation of data-handling practices, and adherence to data governance policies. This is especially important when using ontologies defined by harmonized standards such as the international medical device regulators forum (IMDRF) complaint codes.
- Implement data lifecycle management: Define data lifecycle management practices, including data retention policies, archiving strategies, and end of product life management. Ensure that data is managed throughout its lifecycle in accordance with legal and business requirements.
- Provide training and continuous improvement: Provide training programs for employees involved in data governance to enhance their understanding of policies, procedures, and best practices. Foster a culture of continuous improvement by regularly reviewing and updating data governance practices.
- Leverage technology solutions: Implement data governance tools and technologies to automate data management processes, monitor data quality metrics, enforce policies, and facilitate collaboration among stakeholders.
- Institute a data validation procedure: Employ custom data validation to assess whether the data harmonization process was successful. The validation process will differ depending on the nature of the datasets, whether the harmonization was retrospective or prospective, and the size of the dataset collected and harmonized.
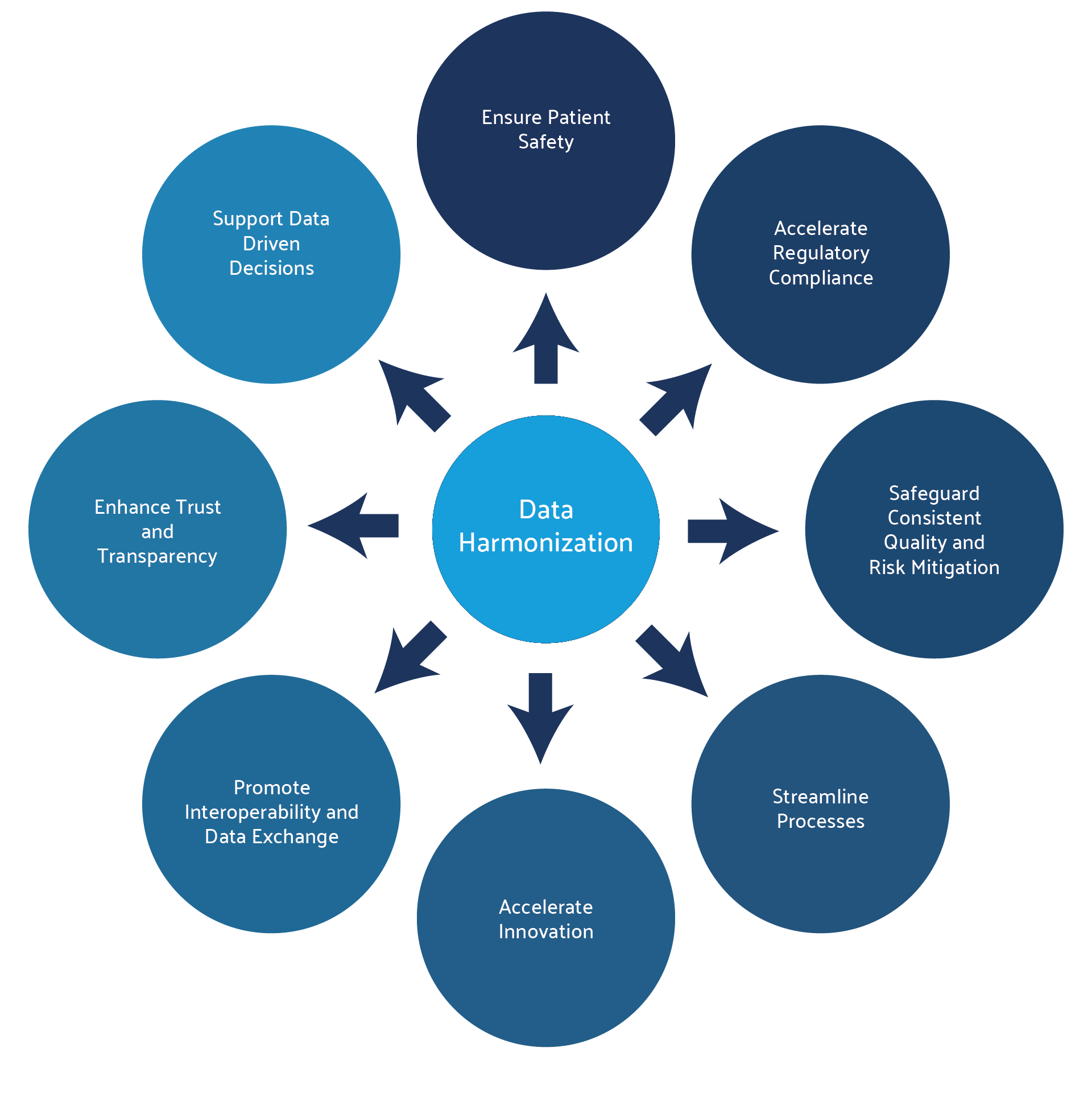
The Case for Data Harmonization at Stryker
This general framework allows organizations to establish a robust data governance structure that supports effective data management, enhances decision-making, mitigates risks, and improves overall operational efficiency and compliance.
A leading example of successful data harmonization is the Trauma and Extremities division at Stryker, led by Sepanta Fazaeli, Clinical Data & Systems Data Lead. 5
In large organizations like Stryker, data is often managed in silos by different functional teams, such as clinical, regulatory, health economics and outcomes research (HEOR), and marketing. Implementing a data harmonization process enabled Sepanta and his team to adopt a holistic approach to evidence data collection, management, analysis, and sharing.
They engaged cross-functional stakeholders to leverage previously extracted literature review data and collaborated with other business units to share standardized workflows and improve overall efficiency.
In the context of clinical evaluation reports, the reuse of templated forms and dropdown menus for reference inclusion and exclusion facilitated efficiency gains across multiple groups and business units.
“In our Trauma and Extremities division, we successfully created standardized forms and templates in DistillerSR, enabling data reuse and enhancing efficiency,” Sepanta explained. “Over the past few months, we have also started to share our best practices and methodologies with other divisions using DistillerSR.”
This collaboration has been highly productive and represents a step towards harmonized practices across the organization. It moves the needle beyond a save-and-store data mindset and establishes a dynamic sharing culture that leverages accessible data lakes, centralized repositories designed to store, process, and secure large amounts of structured or unstructured data.
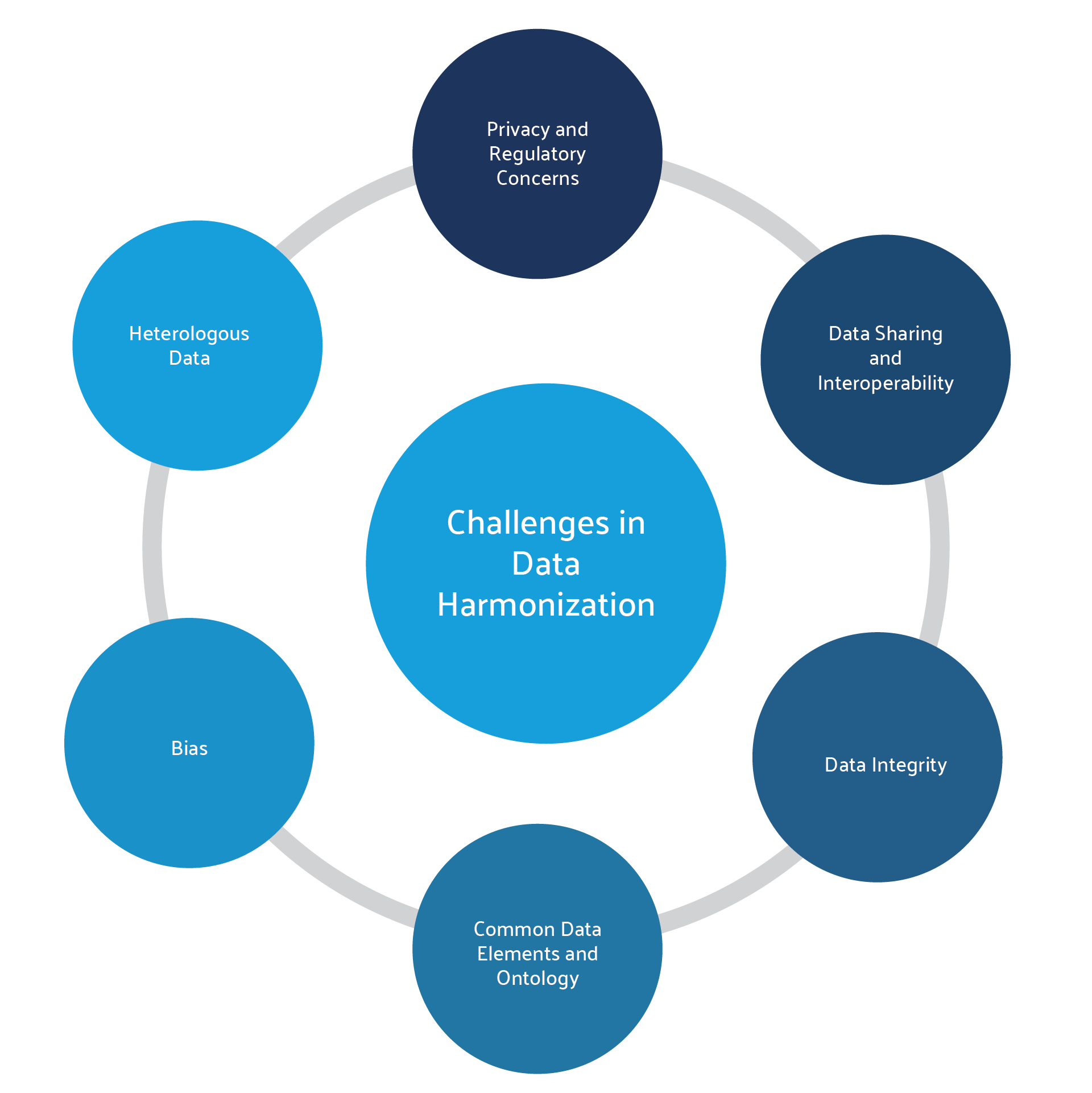
Data Integrity — the Cornerstone of Data Harmonization
Data integrity is key to any harmonization project, as it allows for accuracy, consistency, and reliability over the life cycle of the product. Harmonized data is easier to integrate and aggregate, which is essential to creating comprehensive and standardized evidence repositories. This integration allows for the combination of data from multiple studies, enhancing the breadth and depth of the curated evidence. Ensuring that the data is accurate and unbiased starts with collection of the data, and data integrity processes create a continuous line of traceability, auditability, and trust through analysis, interpretation, and use.
Data integrity systems ensure that the data is intact and unchanged from its source to its destination, without errors, duplicates, corruption, or unauthorized alterations.7 This saves the organization time, resources, and money and allows for solid decision-making.8
All of this is critical to healthcare companies, as shown in the figure 3. Data integrity thus drives better patient outcomes, faster decision-making, and new product development across both pharmaceutical and MedTech companies.3 As noted above, high-quality data also fosters trust with international regulators and stakeholders, enhancing global market opportunities.
Industry-Standard Ontologies and Harmonization Codes in Use
Data harmonization, in contrast to data integration or other analysis techniques, results in a dataset that follows a consistent, cohesive ontology to allow for unified analysis, understandability, and traceability.4
Standardized ontologies establish a common vocabulary for specific domains, such as adverse event reporting. This approach reduces variability and ambiguity in how adverse events are recorded, ensuring that all stakeholders interpret the data in the same way and minimizing the risk of data entry errors.
The result is higher data quality and reliability. In the context of global compliance, standardized ontologies enable the creation of a unified repository for adverse event data that is applicable across various markets, thereby expediting the regulatory submission process.
Ontologies are also critically important for organizations looking to leverage AI or large language models (LLMs). In order for an AI software to correctly analyze data, it need to be trained on the correct databases so that it can detect the correct trends and extract the appropriate information.7 Below is a short list of universal ontologies that can be leveraged during data harmonization.
Harmonization Codes:
- ISO 13485:2016: – the medical device industry’s standard for quality management
Unique Device Identification (UDI) – an identification system for medical devices established by the FDA and adopted by the EU and other countries. - Health Level-7 (HL7) – a framework for the exchange, integration, sharing, and retrieval of electronic health information.
- ISO 14155:2020 – provides requirements for the design, conduct, recording, and reporting of clinical investigations carried out in human subjects.
- ISO 14971:2019 – provides requirements and best practices for risk management throughout the device lifecycle.
- Global Medical Device Nomenclature (GMDN) – a system of internationally agreed-upon generic descriptors for the classification and registration of medical devices.
- European Database on Medical Devices (EUDAMED) – a centralized European database to facilitate regulatory compliance and post-market surveillance.
- EU Medical Device Regulation (MDR) and In Vitro Diagnostic Device Regulation (IVDR) – EU regulations for medical devices and in vitro medical devices that aim to improve device identification and standardize data.
Ontologies:
- Medical Device Ontology (MDO).
- Systematized Nomenclature of Medicine – Clinical Terms (SNOMED CT).
- Medical Dictionary for Regulatory Activities (MedDRA).
Challenges and Opportunities of Data Harmonization
Establishing data harmonization and governance is not without its challenges. First and foremost, the data is often siloed and heterogeneous in nature, meaning that it has already been reviewed and analyzed under different methodologies and must now be reassessed according to a common structure and ontology. The data is often duplicated across multiple systems, and so must be reviewed, cleaned, and deduplicated to ensure data integrity. Teams must then determine how to share the data effectively across the organization while maintaining privacy and security.9 Finally, the organization should weigh the costs and benefits of implementing such a project, since even if harmonization is technically possible, the value of the resulting dataset and analysis may not justify the work it takes to get there.4
For most organizations, these challenges are outweighed by the significant advantages of having clean, complete data that enhances cross-functional analysis and research. Harmonized data offers greater statistical power for analyses compared to individual datasets, making it more valuable to regulatory bodies, payers, healthcare professionals, and patients. Additionally, it allows findings to be generalized across treatments or products based on their mechanism of action. Moreover, the harmonization process can uncover and correct past errors or biases in the original data collection during data integrity audits, thereby improving overall data quality.
Solutions like that integrate multiple datasets – including those from systematic literature reviews and large data systems – are leveraging predictive AI to carry out data hygiene, streamlining the process and making it more efficient for both the governance team and the end user.
Leveraging Technology to Ensure Data Integrity and Harmonization
Good data harmonization generally starts as a manual task, and then can become more automated as the data is categorized and entered into a single system. Skilled data scientists and subject matter experts need to review the data and assign the common data elements, coding, or ontology of choice, and then AI can take over to rapidly carry out sophisticated extraction and analysis. Over time, once a system and a program is agreed upon and implemented, a large part of the overall process can be automated. As more automated systems can be used, the risk of error is reduced as well.8
One of the biggest challenges is finding the correct tools for data harmonization. Introducing new technology in a heavily regulated environment can be challenging. Content-agnostic evidence management platforms can handle diverse data formats and integrate them into a validated workflow. This ability is crucial for data harmonization, which aims to create a consistent data format and structure across various sources.
DistillerSR, for example, seamlessly integrated into Stryker’s technology ecosystem, facilitating efficient data reuse and analysis. This integration has significantly enhanced the team’s ability to derive insights and make evidence-based decisions. As Sepanta noted, “by standardizing our forms and templates in DistillerSR, we have significantly streamlined the literature review processes, from initial screening to data extraction and the creation of clinical evaluation documents for regulatory submissions.” The initial investment and learning curve was quickly offset by dramatic efficiency gains: “We have identified specific families of devices with similarities in labeling and indications, and overlapping search results. This allowed us to reuse extracted data across these similar products. As a result, we have achieved an estimated 70% improvement in efficiency since adopting DistillerSR.”
DistillerSR has been pivotal in advancing Stryker’s enterprise evidence management strategy by addressing critical inefficiencies and compliance challenges. By internally building advanced automation and centralized data management on top of DistillerSR, the team minimized redundancy and reduced costs. The adoption of standardized protocols and templates, along with a comprehensive approach to data collection, sharing, and management has accelerated regulatory compliance. Furthermore, it has fostered a culture of cross-functional collaboration and efficient data reuse.
“I see new opportunities for leveraging DistillerSR across various functions and areas beyond systematic literature reviews,” Sepanta concluded.
“As a domain-agnostic evidence management platform, DistillerSR can be utilized for a wide range of data management purposes, consolidating diverse data types. This capability supports marketing, downstream, and commercial teams by centralizing evidence management and facilitating data reuse. By integrating clinical data with sales and marketing databases, we can accelerate the generation of insights and promote data-driven decision-making.”
References
- Wilkinson MD, Dumontier M, Aalbersberg IJ, et al. The FAIR Guiding Principles for scientific data management and stewardship. Scientific Data. 2016;3(1):160018. doi:10.1038/sdata.2016.18.
- Harmonized scientific data: The key to faster discovery. TetraScience blog. August 27, 2022. https://www.tetrascience.com/blog/harmonized-scientific-data.
- Real-World Evidence. US Food and Drug Administration. 2023.
https://www.ibm.com/blog/mastering-healthcare-data-governance-with-data-lineage. - Cheng C, Messerschmidt L, Bravo I, et al. A general primer for data harmonization. Scientific Data. 2024/01/31 2024;11(1):152. doi:10.1038/s41597-024-02956-3.
- DistillerSR: Stryker Improves Literature Evidence Management Efficiency by 70% with DistillerSR, June 2024.
- Vasileios CP, Dimitrios IF. The pivotal role of data harmonization in revolutionizing global healthcare: A framework and a case study. Connected Health And Telemedicine. 2024;3(2):300004.
- Przybycień G. Data integrity vs. data quality: Is there a difference? IBM blog. July 13, 2023. https://www.ibm.com/think/topics/data-integrity-vs-data-quality.
https://hbr.org/2016/09/bad-data-costs-the-u-s-3-trillion-per-year - Cote C. What is data integrity and why does it matter? HBS Online. February 4, 2021. https://online.hbs.edu/blog/post/what-is-data-integrity.
- Sayyed N. Data harmonization: Steps and best practices. Datavid blog. September 7, 2023. https://datavid.com/blog/data-harmonization.