Although artificial intelligence technology is advancing at an incredible pace, it’s still common for researchers to hesitate when it comes to implementing it into their systematic review processes. Why?
For many researchers, it comes down to uncertainty about the technology and aversion to risks that could compromise the validity of the review. However, it’s important to note that pragmatic adoption of AI in systematic review processes can be relatively low-risk.
When looking at the minimum accuracy target for an AI, we should consider that the gold standard for reviewing is not without flaws. Accepting AI error rates that are on par with human error rates is often sufficient and does indeed save time and resources in the review process. Past research has concluded that the human reviewers are not perfect. The key is to assess the acceptable risk you are willing to take and implement AI in a way that won’t cause catastrophe if a mistake is made.
Here are our top 3 low-risk, big reward ways to use AI in your literature review process:
1. Find Relevant Records Faster with Reprioritization
It’s important for teams to efficiently identify their included articles since there are often thousands or even tens of thousands of articles to screen. One AI-powered solution for speeding up this step of the systematic review process is automatic reference reprioritization.
This is a DistillerSR AI feature that runs in the background, using natural language processing (NLP) to learn from articles you have already screened. As it learns from the reviewer’s behavior, it re-ranks the references by likelihood of inclusion or relevance, presenting you with records that are most likely to be relevant.
Automatic reference reprioritization is particularly helpful in instances where you know there is a lot of evidence on the topic, and there are a lot of articles to get through. It creates efficiency throughout the screening process and enables researchers to move onto other parts of the review, such as obtaining full-text documents, data extraction, and more, sooner. It’s low-risk because you are still screening the way you normally would, but with most of the relevant articles loaded to the front of your screening list.
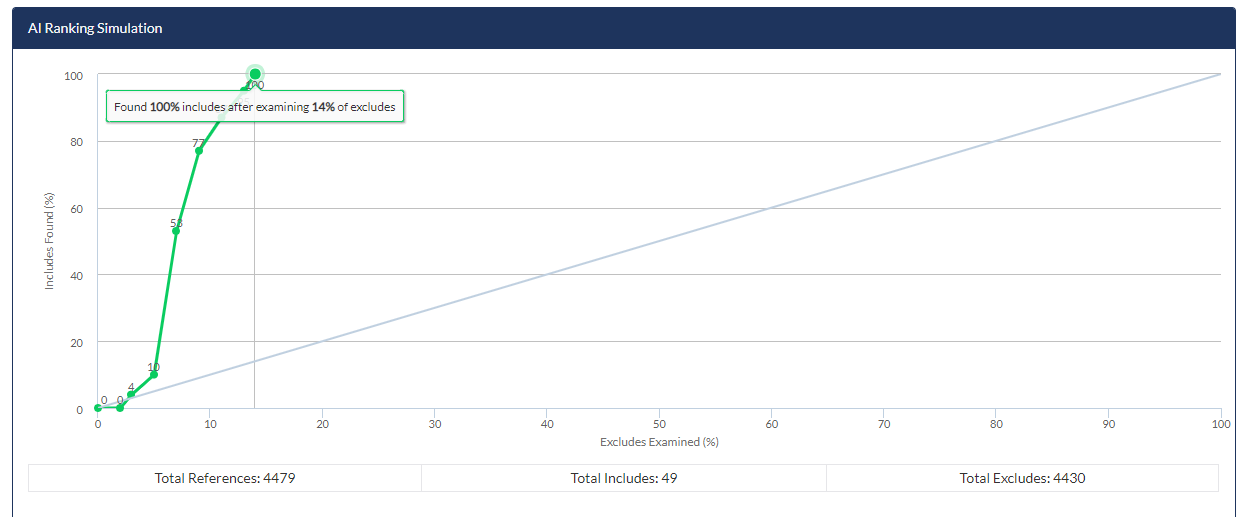
2. Predict How Far Along You Are in the Screening Process
The main reasons for researchers to use AI in their literature review process are to save time and improve efficiency. However, it might be difficult to get a sense of these metrics in action. One of the best ways to actually see how the AI is impacting your review is by using a predictive reporting tool.
In DistillerSR, the predictive reporting tool provides you with:
- An estimate of what proportion of relevant studies you have identified
- How often your team is finding relevant studies
- Relative likelihood of relevance for records that are yet to be reviewed
Additionally, if you want to see how AI could have helped in a past project, you can use DistillerSR’s AI Simulation tool to retrospectively examine a past project, giving you more insight on the use of AI in your current projects.
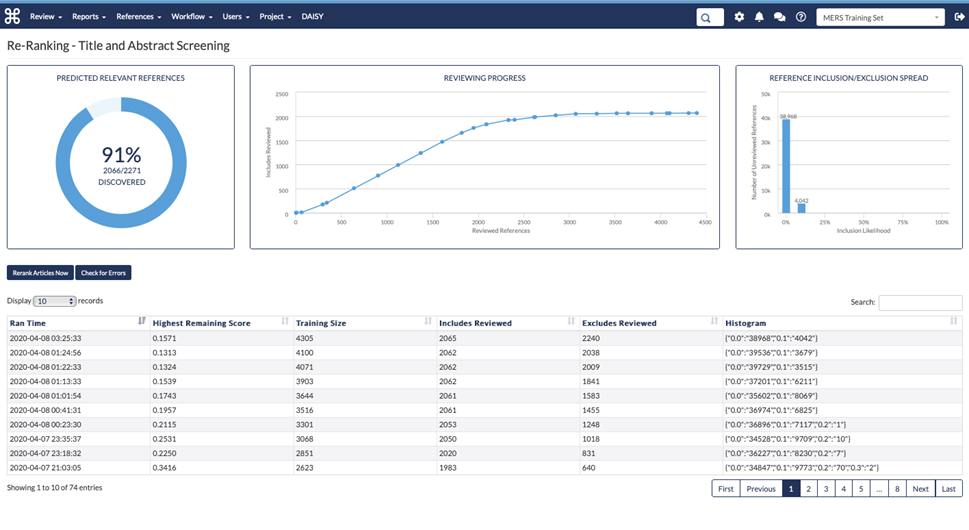
3. Identify Errors
For many research teams, accidentally excluding a relevant article is one of the most detrimental errors to make. As we have previously mentioned in past blogs, humans are not perfect when it comes to screening, and mistakes can go unnoticed, especially when using single reviewer screening methods.
With AI, you can easily audit your work, helping you identify potential errors in your screening quickly and easily. For example, the AI can automatically review your excluded records to identify any that closely resemble included records. The risk factor here is low because the reviewer will ultimately decide if the reference should indeed be included. It enables reviewers to double or even triple check their work, reducing errors and increasing confidence.
Ultimately, there is no magic button to press and complete a systematic review. But when AI is implemented pragmatically, it has many benefits that are relatively low risk. The key is assessing your organization’s individual risk tolerance and building safeguards into your process. We always recommend using AI in conjunction with qualified and trained researchers.
With tools like automatic reference reprioritization, AI audit and simulation, and predictive reporting, as well as DistillerSR’s DIY classifiers, research teams can better understand how the AI is performing for each individual project or circumstance. This, in turn, helps them make informed decisions about the best ways to leverage the AI based on unique topics, risk tolerances, or stakeholder needs.