White Paper
Purpose-built GenAI for Literature ReviewsExecutive Summary
The surge in scientific literature presents a challenge to researchers who must produce accurate, high- quality literature reviews within an increasingly complex regulatory landscape. This rising volume, combined with labor shortages and intensifying cost pressures, makes the need for efficient, reliable literature review solutions more critical than ever.
To date, AI has successfully automated certain aspects of the review process, notably reference screening. However, the next frontier—data extraction—remains one of the most time-consuming, error-prone stages. Data extraction is essential, yet traditionally manual and prone to reviewer fatigue. Generative AI (GenAI) offers new possibilities with its capacity to process large volumes of information and generate responses to complex questions. Yet, GenAI alone has limitations: it lacks crucial ingredients for performing accurate, repeatable, transparent, auditable evidence extraction, especially while respecting copyright and protecting sensitive data. Consequently, regulators require that human reviewers stay “in the loop” of evidence extraction.
DistillerSR’s latest capability, Smart Evidence Extraction™ (SEE), successfully addresses these challenges. SEE uses a composite of AI technologies, applying deterministic AI techniques to leverage the strengths of GenAI while mitigating its weaknesses. Designed to alleviate reviewer fatigue while maintaining complete control and accuracy, SEE keeps human reviewers in charge, providing suggested data extraction with full transparency.
Each piece of extracted information is directly linked to its source document, allowing reviewers to verify its origin and accuracy easily. This approach ensures compliance with copyright and regulatory standards and offers unprecedented transparency in evidence extraction.
SEE is built on the principles of responsible AI, embedding fairness, accountability, and transparency (FAccT) into every aspect of its design. This proprietary model eliminates the risk of GenAI’s “hallucinations” and probabilistic outputs, which can undermine trust in fully automated systems. Instead, SEE emphasizes trustworthiness through clear provenance, secure in-house data handling, and a human-centered approach that supports reviewers in making precise, validated decisions.
By integrating GenAI into a responsible, auditable framework, DistillerSR’s Smart Evidence Extraction™ allows organizations to manage literature reviews more effectively and compliantly.
With SEE, reviewers can focus on high-value work, reduce fatigue, and meet the rising demand for faster, more accurate reviews.
Download the PDF of this Brief
Introduction
In the broadening landscape of literature review software, evidence extraction has so far resisted automation. Reviewers spend a significant amount of time on this step, making it a leading contributor to fatigue. This is compounded by the rate of published literature, with 3 million scientific articles published in English every year, and the volume growing by 8% to 9% annually.1
Meanwhile, organizations want better outcomes for patients and customers, and more timely information to make business decisions. These goals are complicated by skill shortages,2 copyright issues, high rates of human error,3 and increasingly stringent regulatory standards.
Generative artificial intelligence (GenAI) is a recent innovation that promises to revolutionize evidence extraction, letting reviewers focus their skills more efficiently and giving organizations more flexibility in resourcing.
GenAI’s capacity to process large amounts of information and produce answers to arbitrary questions is impressive. But GenAI by itself is not yet a proven, trustworthy solution for literature reviews4: it lacks crucial ingredients for performing accurate, repeatable, transparent, auditable evidence extraction, especially while respecting copyright and protecting sensitive data. Consequently, regulators require that human reviewers stay “in the loop” of evidence extraction.5
What’s needed, is a technology that harnesses GenAI to extract evidence while staying inside the guardrails of literature reviews. This would reduce reviewer fatigue and support organizations with their regulatory and business demands. It would also open new opportunities for the future in other areas that need to extract and structure data.
DistillerSR’s AI Track Record
DistillerSR is both an early innovator and the leader in applying AI to literature reviews. Healthcare, public sector, and academic organizations around the world, including the largest pharmaceutical and medical devices companies, use DistillerSR.
In 2008, DistillerSR introduced literature review automation with collaboration features that let multiple reviewers concurrently work in a single review, speeding up completion time.
In 2018, DistillerSR released the AI Toolkit, the first iteration of AI in literature review software, featuring AI Error Checking to detect for screening errors made by human reviewers. Since then, DistillerSR has added other AI-enhanced automation that has been repeatedly shown to reduce reviewer fatigue, accelerate the review process, and improve data quality.6
Until recently, DistillerSR’s AI-enabled capabilities used deterministic AI to help reviewers screen, highlight, and categorize references as well as prioritize literature. The remaining, and perhaps the most tedious, step in the literature review workflow is evidence extraction. This required a different approach.
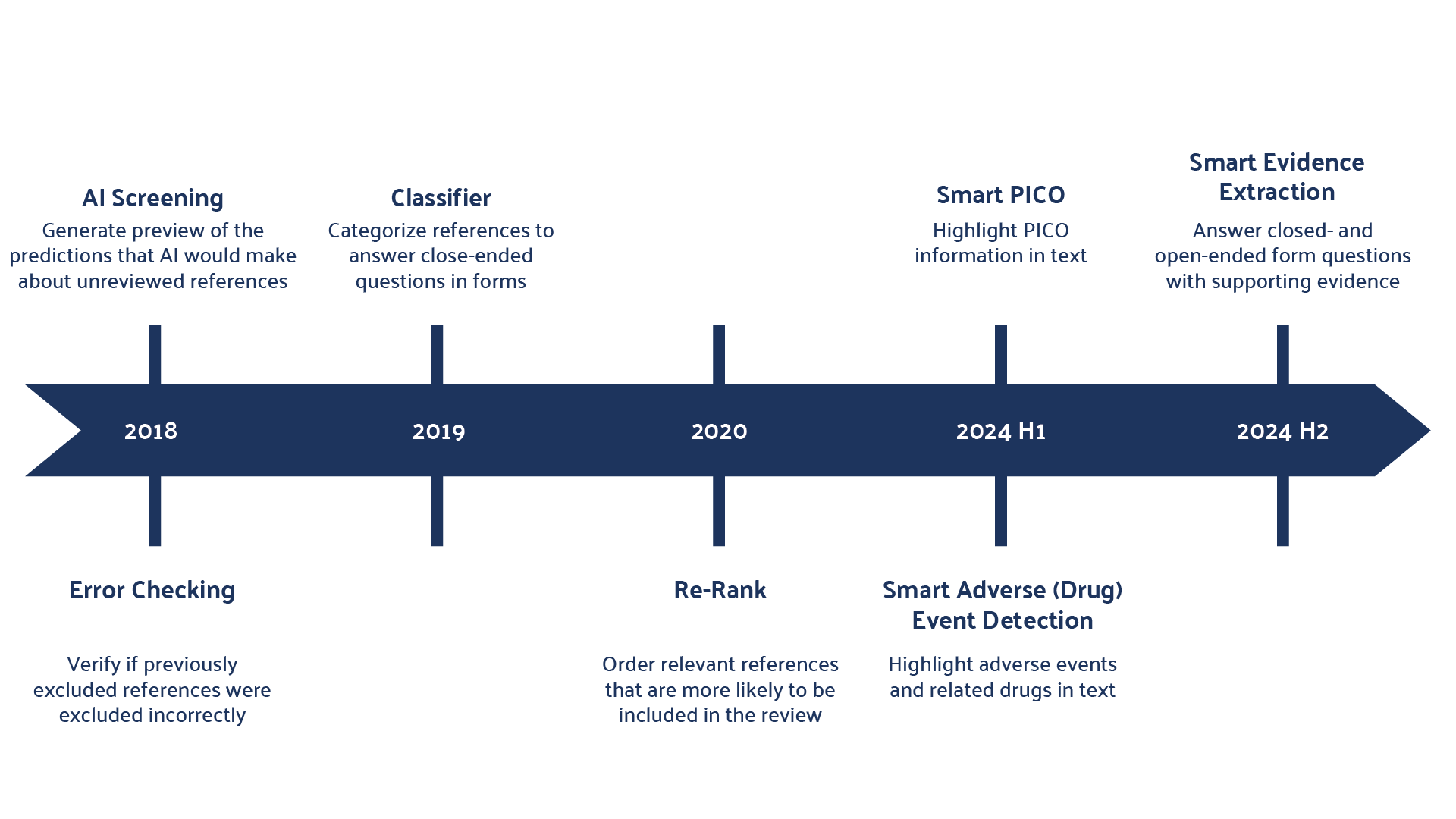
Automating Evidence Extraction
It’s reasonable to expect that automating evidence extraction would have definite benefits. Reviewers are a limited resource and highly specialized in their domain. Manual evidence extraction takes away time and effort that could be better spent on higher value work.
Automating evidence extraction faces technical obstacles:
- Accuracy: The reviewer must be able to continue to answer protocol questions with the same or increased accuracy level.
- Copyright and sensitive information: Automated extraction must not breach copyright laws or expose sensitive information in the reviewed literature.
However, the biggest obstacle is trust. Organizations and regulators must have confidence in the final review data.
Automated evidence extraction must efficiently generate reproducible answers with supporting evidence for the reviewer to validate. Its operation must be transparent and auditable.
A successful approach would take into account the factor analysis of information risk (FAIR) framework to center GenAI on the ethical and research requirements of the organization – a methodology applied by DistillerSR in the development of their latest AI-enabled capability: Smart Evidence Extraction (SEE)™.
The Promise of Generative AI
OpenAI’s ChatGPT chatbot has shown how GenAI can respond to complex prompts with mostly correct responses on a wide variety of topics.7 According to the Gartner Hype Cycle analysis,8 the early popularity of GenAI has already crested the peak of inflated expectations and is only recently climbing out of the trough of disillusionment. GenAI providers are constantly implementing solutions to work around the limitations of this technology.
Despite these limitations, the potential is still evident. With the leaps in innovation in large language models (LLMs) and GenAI, automated evidence extraction seems within reach.
For the reviewer, GenAI’s bold promise is to dramatically accelerate the research process while reducing the fatigue that comes with a growing workload.
The possibility of covering more literature with greater accuracy is obviously appealing. And GenAI has the potential to reduce bias, too: imagine a tool that lets the reviewer confirm their own answers against the machine’s.
For organizations, GenAI promises faster completion rates for literature reviews, broader coverage, and better outcomes for patients and customers. Businesses also expect quicker time to market and more timely business decisions.
The long-term potential of GenAI is especially exciting. Fundamentally, GenAI is about converting large volumes of unstructured data to validated, structured information. This has clear value and applicability for systematic literature review. It also makes GenAI applicable for other tasks, like data science and AI training.
The Limitations of Vanilla AI
Commercial, off-the-shelf GenAI services, also known as “vanilla AI,” are impressive. But using vanilla AI for literature reviews quickly reveals that there is no “easy” button. Vanilla AI is purposely generalized for broad applications, making it unsuitable for literature reviews out of the box.
Perhaps more troubling, there is little transparency into or auditability of the sources used in its training and fine-tuning of data, raising concerns among copyright holders. For these reasons, no regulator accepts AI-generated reviews.
Vanilla AI is intentionally, inherently generative and probabilistic. For some applications, the guarantee of an answer irrespective of the confidence it inspires is considered an asset. But for literature reviews, it’s a liability.
The most immediate consequence of GenAI’s behavior is its potential for hallucination and suggesting different responses for the same prompt.
This makes prompt engineering a key skill, requiring the reviewer to be keenly aware of vanilla AI’s limitations and capabilities. Further, its responses must be mediated by a human to get deterministic results, ironically adding to reviewer fatigue.
The corpus itself, on which vanilla AI depends for training and fine-tuning, is drawn from multiple sources, including the prompts and responses of all users of the AI model. With little transparency into or ability to audit of this material, there are valid, unresolved concerns about these programs’ treatment of sensitive information, including intellectual property, copyrighted material, and patient information.9
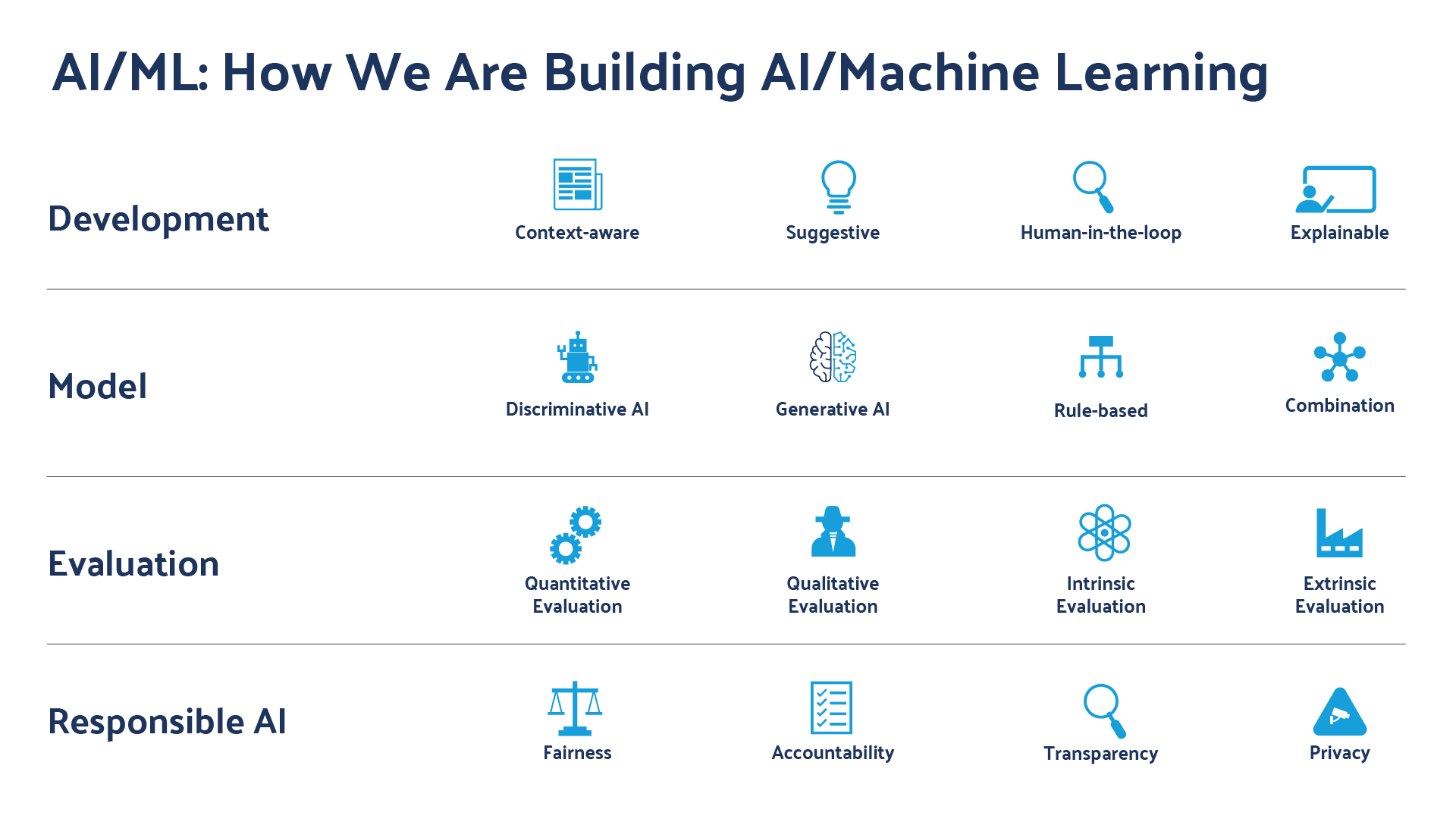
The DistillerSR Approach
DistillerSR’s latest enhancement to the literature review workflow, Smart Evidence Extraction (SEE), is a major step toward automating evidence extraction. SEE’s goal is to assist the reviewer in producing accurate, grounded evidence while reducing the strain that comes with reviewing large volumes of references and data. Its design and implementation adhere to the principles of responsible AI, namely fairness, accountability, and transparency (FAccT).
By design, DistillerSR’s SEE is a suggestive AI for evidence extraction, assigning the reviewer as the authority on the extracted evidence. SEE’s suggestions contain links to the supporting evidence in the original source document, maintaining complete transparency and auditability in terms of the provenance of every cell of extracted data.
The implementation of SEE uses a composite of AI technologies, applying deterministic AI techniques to leverage the strengths of GenAI while mitigating its weaknesses.
To eliminate hallucination, SEE uses a mix of GenAI techniques and SEE’s context awareness. Instead of generating its responses only from the broad data of its pretraining, context awareness focuses the GenAI’s responses on the literature being reviewed.
Furthermore, copyrighted and other sensitive information are protected because all of SEE’s infrastructure resides inside the DistillerSR environment, with no information sent to outside services or LLMs.
Human-in-the-loop remains a fundamental tenet of valid data extraction. To ensure it, SEE requires reviewers to either accept or reject SEE’s suggested answers.
SEE highlights supporting evidence in the literature and provides attention maps, natural language explanations, and a confidence score. These features also have the potential to reduce bias, by giving the reviewer another interpretation of the reference material.
The final step of SEE allows the reviewer to link the evidence from the source document to the accepted answer, which significantly improves the auditability of, and ultimately the trust in the review output.
With SEE, reviewers reduce the time spent on evidence extraction while maintaining quality.
SEE also opens new opportunities after the review is completed. Organizations can leverage this structured, validated data for reuse in other literature reviews, data analysis and AI training. DistillerSR’s add-on module CuratorCR enables automated data reuse, curation, and sharing, supporting organizational efforts towards data harmonization and data governance.
The Future State of Automated Evidence Extraction
DistillerSR’s SEE is a no-code evidence extraction solution that enables reviewers and organizations extract and reuse accurate data that is validated, traceable, and trustworthy.
This viable, reliable implementation of GenAI puts organizations in a position to consider new applications beyond evidence extraction. Before that can happen, however, industry and regulators need to trust automated evidence extraction. To address this need, DistillerSR is leading a six-country consortium to specify a standard for automated data extraction from scientific literature. The artificial intelligence data extraction for scientific literature (AIDESL) consortium project will develop commercially viable products that establish standards for ethical, large-scale, accurate, validated, and auditable automated evidence extraction.10 The expected start date for the project is early 2025.
Conclusion
Evidence extraction leaves reviewers fatigued and slows the time to market. Automating evidence extraction has been challenging, with technical complications and a lack of regulatory acceptance.
With the introduction of DistillerSR’s Smart Evidence Extraction, human reviewers have a trusted companion in their literature review journey. SEE casts GenAI in a role for evidence extraction that gives timely, accurate, reproducible, validated, and trustworthy results. The evolution in this area upholds the promise of AI and builds a pathway that the AIDESL project aims to fulfill.
References
- Johnson R, Watkinson A, Mabe M. The STM Report: An overview of scientific and scholarly publishing (Fifth Edition). The Hague: International Association of Scientific, Technical and Medical Publishers. 2018. https://stm-assoc.org/wp-content/uploads/2024/08/2018_10_04_STM_Report_2018-1.pdf.
- Randstad Enterprise. 2024 talent trends research: Life sciences and pharma. July 26, 2024.
https://www.randstadenterprise.com/insights/life-sciences-pharma/global-talent-trends-life-sciences-pharma/ - Wang Z, Nayfeh T, Tetzlaff J, O’Blenis P, Murad MH. Error rates of human reviewers during abstract screening in systematic reviews. PLoS One 2020;15(1). https://doi.org/10.1371/journal.pone.0227742.
- Abd-alrazaq A, AlSaad R, Alhuwail D, Ahmed A, Healy P, Latifi S, Aziz S, Damseh R, Alabed Alrazak S, Sheikh J. Large language models in medical education: Opportunities, challenges, and future directions. JMIR Med Educ 2023;9:e48291. https://mededu.jmir.org/2023/1/e48291.
- National Institute for Health and Care Excellence. Use of AI in evidence generation: NICE position statement. August 15, 2024. https://www.nice.org.uk/about/what-we-do/our-research-work/use-of-ai-in-evidence-generation–nice-position-statement.
- DistillerSR. DistillerSR AI. 2024. https://www.distillersr.com/products/distillersrai.
- Balhorn LS, Weber JM, Buijsman S, Hildebrandt JR, Ziefle M, Schweidtmann M. Empirical assessment of ChatGPT’s answering capabilities in natural science and engineering. Sci Rep 2024;14, 4998. https://doi.org/10.1038/s41598-024-54936-7.
- Staab R, Vero M, Balunović M, Vechev M. Beyond Memorization: Violating Privacy Via Inference with Large Language Models. 2024. https://doi.org/10.48550/arXiv.2310.07298.
- ITEA4. AIDESL. Fully Automated AI Data Extraction from Scientific Literature. 2023. https://itea4.org/project/aidesl.html.